Neuromorphic Hardware Guide
Explore cutting-edge neuromorphic chips and architectures, featuring innovative designs and advanced neural processing technologies.
- Home /
- Neuromorphic Computing /
- Neuromorphic Hardware Guide
Neuromorphic Hardware: A Comprehensive Guide
Delve into the evolution of neuromorphic hardware, uncovering its rich history, detailed specifications, and the brilliant developers behind groundbreaking projects. Discover key milestones, technical intricacies, and the visionary minds shaping the future of intelligent computing.
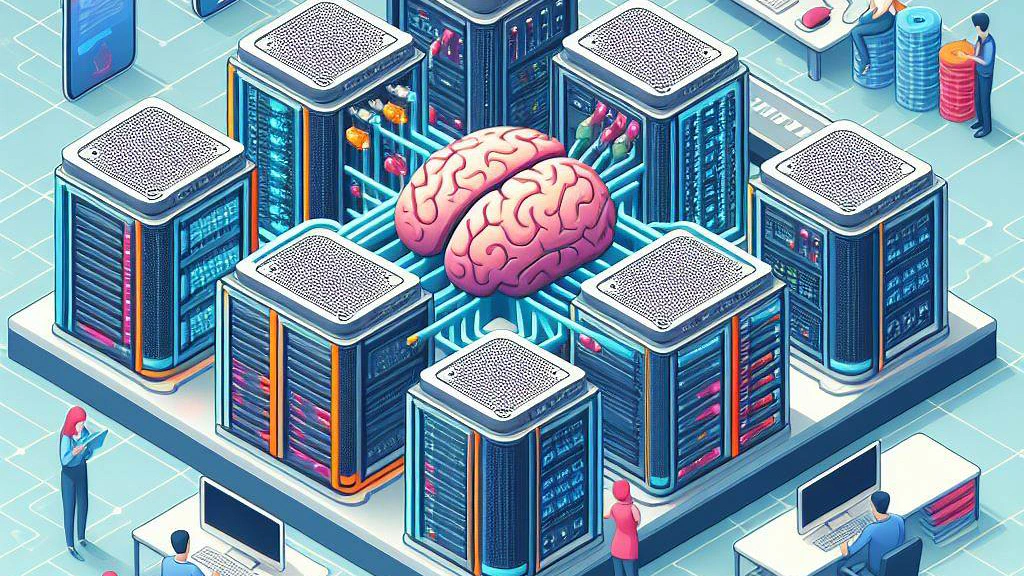
Find specifications, papers, and development details:
- 2024
Spiking Neural Processor T1 by InnateraView DetailsThe Spiking Neural Processor T1 is Innatera's ultra-low-power neuromorphic microcontroller SoC for real-time intelligence close to the sensor. It integrates a spiking neural network accelerator, a convolutional neural network accelerator and a RISCV core. T1 targets applications in battery-powered, power-limited and latency-critical devices.
- 2022
Akida - BrainChipView DetailsBrainChip's Akida is an ultra-low-power neuromorphic processor inspired by the brain's neural architecture. It accelerates complex AI at the edge through event-based processing, on-chip learning abilities, and support for advanced neural networks like CNNs, RNNs & custom Temporal Event-based Nets.
BrainScaleS 2 - Universität HeidelbergView DetailsThe BrainScaleS-2 is an accelerated spiking neuromorphic system-on-chip integrating 512 adaptive integrate-and-fire neurons, 212k plastic synapses, embedded processors, and event routing. It enables fast emulation of complex neural dynamics and exploration of synaptic plasticity rules. The architecture supports training of deep spiking and non-spiking neural networks using hybrid techniques like surrogate gradients.
ReckOn - Charlotte FrenkelView DetailsReckOn demonstrates, for the first time, end-to-end on-chip learning over second-long timescales (no external memory accesses, no pre-training). It is based on a bio-inspired alternative to backpropagation through time (BPTT), the e-prop training algorithm, which has been modified to reduce the memory overhead required for training to only 0.8% of the equivalent inference-only design. This allows for a low-cost solution with a 0.45-mm² core area and a <50-µW power budget at 0.5V for real-time learning in 28-nm FDSOI CMOS, which is suitable for an always-on deployment at the extreme edge. Furthermore, similarly to the brain, ReckOn exploits the sensor-agnostic property of spike-based information. Combined with code-agnostic e-prop-based training, this leads to a task-agnostic learning chip that is demonstrated on vision, audition and navigation tasks.
Speck - SynSenseView DetailsSpeck is a fully event-driven neuromorphic vision SoC. Speck is able to support large-scale spiking convolutional neural network (sCNN) with a fully asynchronous chip architecture. Speck is fully configurable with the spiking neuron capacity of 328K. Furthermore, it integrates the state-of-art dynamic vision sensor (DVS) that enables fully event-driven based, real-time, highly integrated solution for varies dynamic visual scene. For classical applications, Speck can provide intelligence upon the scene at only mWs with a latency of 3.36us for a single event processed by a 9 layer network.
Xylo - SynSenseView DetailsXylo is a 28nm 1000 neuron digital spiking neural network inference chip optimized for ultra-low power edge deployment of trained SNNs, with a flexible architecture to map various network topologies.
- 2021
Loihi 2 - IntelView DetailsLoihi 2 is Intel's latest neuromorphic research chip, implementing spiking neural networks with programmable dynamics, modular connectivity, and optimizations for scale, speed, and efficiency. Early research demonstrates promise for low-latency intelligent signal processing.
SpiNNaker 2 - University of DresdenView DetailsThe SpiNNaker2 chip houses 153 ARM cores with 19MB on-chip SRAM, 2GB DRAM, and dedicated Machine Learning (e.g., MAC) and Neuromorphic (e.g., Exp/Log) accelerators. Manufactured in 22nm FDSOI, it employs Adaptive Body Biasing (ABB) in a Forward Body Bias (FBB) configuration, as well as DVFS for adaptive near-threshold operation down to 0.5V, enabling a 10x increase in neural simulation capacity per watt over SpiNNaker1.
- 2019
DynapCNN - SynSenseView DetailsThe DynapCNN is an ultra-low power, event-driven neuromorphic processor chip for spiking neural networks that achieves sub-milliwatt computation using in-memory techniques. With 1M neurons, it can implement convolutional network models like LeNet and ResNet, interfacing directly to sensors like DVS cameras for low-latency, always-on vision applications.
Odin by Charlotte FrenkelView DetailsThe ODIN 256-neuron 64k-synapse neuromorphic processor highlights how design constraints on the synapses can be released by offloading most synaptic computations at the neuron level. All synapses embed spike-driven synaptic plasticity (SDSP), while neurons are able to phenomenologically reproduce the 20 Izhikevich behaviors of cortical spiking neurons. At the time of publication, ODIN demonstrated the highest synaptic density, and the lowest energy per synaptic operation among digital designs. ODIN was fabricated in 28nm CMOS and can be prototyped in small FPGAs.
DYNAP-SE2 - Institute of NeuroinformaticsView DetailsThe DYNAP-SE2 is a configurable, mixed-signal neuromorphic chip featuring 1024 neurons, 65k plastic synapses, specialized dendrites, low-latency event routing, and multi-timescale adaptation dynamics. This enables real-time prototyping of biologically inspired spiking neural networks for ultra-low power edge processing.
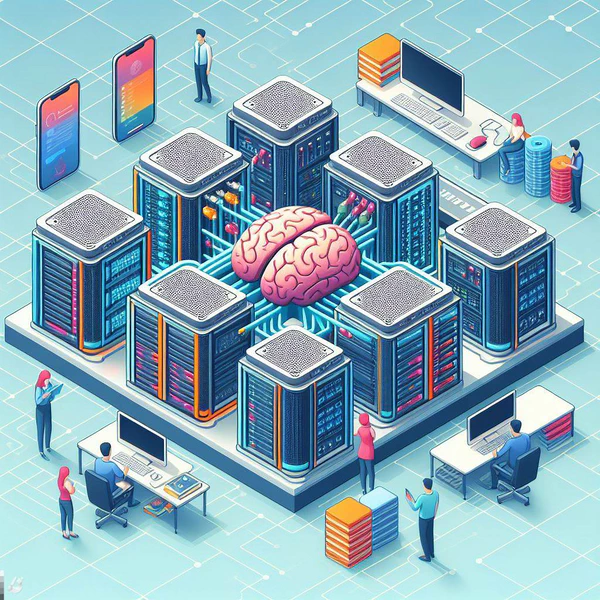
Current Landscape and Future Prospects (2020s and Beyond)
In the current era, neuromorphic hardware continues to evolve rapidly. Various research projects and commercial ventures focus on enhancing the efficiency, scalability, and applicability of neuromorphic systems. The field holds promise for addressing complex tasks in artificial intelligence, neuromorphic computing, and brain-machine interfaces.
Advancements in Spiking Neural Networks (2010s)
The 2010s marked significant advancements in spiking neural networks (SNNs) and event-driven computing. These developments enabled more efficient and power-aware neuromorphic hardware designs. Initiatives like the Human Brain Project and the development of specialized neuromorphic chips, such as IBM's TrueNorth, showcased the potential of this technology.
The Birth of True Neuromorphic Chips (1990s-2000s)
In the 1990s and 2000s, the development of true neuromorphic chips accelerated. Carver Mead's pioneering work laid the foundation for creating circuits that emulate the brain's synapses and neurons. Research institutions and companies began exploring neuromorphic architectures for specialized applications like pattern recognition and sensory processing.
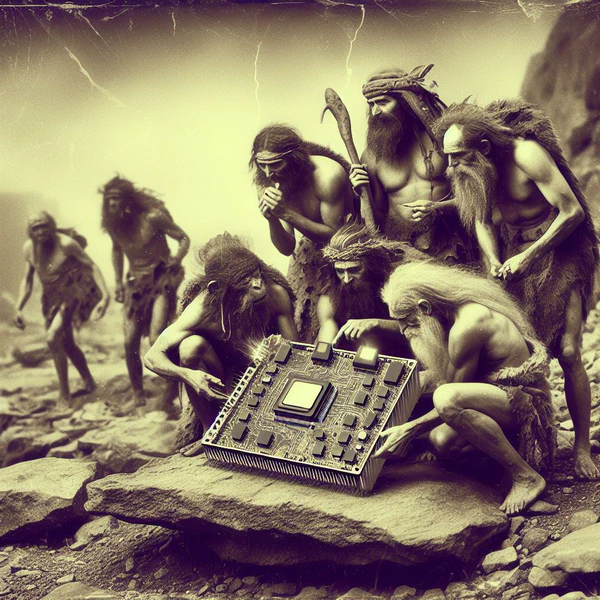
Early Concepts (1940s-1980s)
The roots of neuromorphic hardware can be traced back to early computational neuroscience efforts in the 1940s. Researchers explored mimicking the brain's structure and functionality. In the 1980s, the concept of neuromorphic engineering gained momentum, focusing on hardware implementations inspired by the brain's neural networks.
Discontinued or Unsupported
Loihi - Intel
Loihi 1 is Intel's advanced neuromorphic chip, designed to mimic brain-like processing, enabling efficient, adaptive machine learning applications.
NeuroGrid (BrainDrop) - Stanford
Neurogrid is a specialized neuromorphic hardware system that enables real-time simulation of neural models with up to 1M neurons & 8B synapses using low-power mixed-signal silicon neuron circuits arranged in 16 chips & interconnected via multicast routing.
ROLLS - INI
ROLLS is a reconfigurable neuromorphic chip with 256 silicon neurons and 128K plastic synapses that implements spike-timing learning rules. It allows emulation of neural systems with adaptive behaviors using analog neuron/synapse circuits with added digital configuration logic for flexibility.
Tianjic - Tsinghua University
Tianjic supports both spiking and non-spiking models. Its motivation is to enable hybrid networks that blend biological plausibility from neuroscience with predictive accuracy from deep learning.
TrueNorth - IBM
TrueNorth is a 5.4B transistor, 4096 core, 1M neuron, 256M synapse neurosynaptic chip implemented in 28nm. Through a mixed async-sync design & custom toolflow, it achieves 58GSOPS & 400GSOPS/W efficiency while running neural networks in 65mW real-time.
Glossary
- Spiking Neural Network (SNN): A type of artificial neural network that closely models the spiking behavior of biological neurons, utilizing discrete spikes or pulses of activity for information processing.
- Event-Driven Computation: A computing paradigm where processing occurs in response to specific events or stimuli, allowing for energy-efficient operation and asynchronous communication between components.
- Synapse: The functional connection between two neurons or between a neuron and another cell, where signals are transmitted through chemical or electrical means.
- Plasticity: The ability of synapses to strengthen or weaken over time, a key feature in neuromorphic hardware that enables learning and adaptation.
- Spike-Timing-Dependent Plasticity (STDP): A type of synaptic plasticity in which the timing of neural spikes influences the strength of the synapse, essential for learning and memory in neuromorphic systems.
- Memristor: A resistor with memory, a key component in neuromorphic hardware that can store and process information, mimicking the synaptic plasticity found in biological systems.
- Neuromorphic Chip: A specialized hardware component designed to implement neuromorphic computing principles, often featuring a large number of simple, interconnected processing units.
- Neuromorphic Engineering: The interdisciplinary field that combines principles from neuroscience, physics, computer science, and engineering to design and build brain-inspired computing systems.
- Event-Based Sensor: A sensor that captures and transmits information in an event-driven manner, aligning with the principles of neuromorphic hardware for efficient and low-latency data processing.
- SpiNNaker (Spiking Neural Network Architecture): A neuromorphic computing platform designed for simulating large-scale spiking neural networks, with a focus on real-time processing and parallel communication.